Machine Learning and Deep Learning in Genetics and Genomics
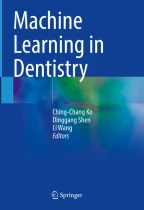
In this chapter, we introduce various machine learning (ML) methods and deep learning (DL) algorithms, commonly adopted in genomics data analysis. We begin with a general introduction of genomics data and present a multi-omics study investigating early childhood oral health. We then review statistical methods and ML/DL methods and their application in genomics data analysis that include the following aspects: (1) association between genetic markers, mostly single nucleotide polymorphisms (SNPs), and complex diseases or traits in genome-wide association studies (GWAS), (2) copy number variation (CNV), and single nucleotide variant (SNV) calling in whole genome sequencing (WGS) or whole exome sequencing (WES) data of tumor samples, (3) association between DNA methylation status and phenotypes, which are commonly referred to as epigenome-wide association studies (EWAS), (4) analysis of genome-wide high-throughput chromosome conformation capture (Hi-C) data, (5) inference related to transcription factor binding sites (TF), and (6) single-cell RNA-seq data analysis. To complete the review, we present the results of a systematic review of the machine learning landscape in oral diseases. We conclude with a discussion of potential future applications of ML/DL in genetics and genomics in oral health.
This is a preview of subscription content, log in via an institution to check access.
Access this chapter
Subscribe and save
Springer+ Basic
€32.70 /Month
- Get 10 units per month
- Download Article/Chapter or eBook
- 1 Unit = 1 Article or 1 Chapter
- Cancel anytime
Buy Now
Price includes VAT (France)
eBook EUR 74.89 Price includes VAT (France)
Softcover Book EUR 94.94 Price includes VAT (France)
Hardcover Book EUR 137.14 Price includes VAT (France)
Tax calculation will be finalised at checkout
Purchases are for personal use only
Similar content being viewed by others
RNA Sequencing and Genetic Disease
Article 21 June 2016
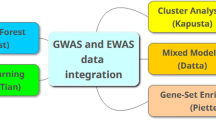
Data mining and machine learning approaches for the integration of genome-wide association and methylation data: methodology and main conclusions from GAW20
Article Open access 17 September 2018
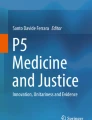
The Origin of Personalized Medicine and the Systems Biology Revolution
Chapter © 2017
References
- McCulloch WS, Pitts W. A logical calculus of the ideas immanent in nervous activity. Bull Math Biophys. 1943;5(4):115–33. ArticleGoogle Scholar
- Park WJ, Park J-B. History and application of artificial neural networks in dentistry. Eur J Dent. 2018;12(04):594–601. ArticlePubMedPubMed CentralGoogle Scholar
- Lin E, Lane H-Y. Machine learning and systems genomics approaches for multi-omics data. Biomarker Res. 2017;5(1):2. ArticleGoogle Scholar
- Krizhevsky A, Sutskever I, Hinton GE. Imagenet classification with deep convolutional neural networks. Adv Neural Informn Proc Syst. 2012;25:1097–105. Google Scholar
- Hung M, Voss MW, Rosales MN, Li W, Su W, Xu J, et al. Application of machine learning for diagnostic prediction of root caries. Gerodontology. 2019;36(4):395–404. ArticlePubMedPubMed CentralGoogle Scholar
- Liu Z, Liu J, Zhou Z, Zhang Q, Wu H, Zhai G, et al. Differential diagnosis of ameloblastoma and odontogenic keratocyst by machine learning of panoramic radiographs. Int J Comput Assist Radiol Surg. 2021;16(3):415–22 ArticlePubMedPubMed CentralGoogle Scholar
- Abdalla-Aslan R, Yeshua T, Kabla D, Leichter I, Nadler C. An artificial intelligence system using machine-learning for automatic detection and classification of dental restorations in panoramic radiography. Oral Surg Oral Med Oral Pathol Oral Radiol. 2020;130(5):593–602. ArticlePubMedGoogle Scholar
- Xie X, Wang L, Wang A. Artificial neural network modeling for deciding if extractions are necessary prior to orthodontic treatment. Angle Orthod. 2010;80(2):262–6. ArticlePubMedPubMed CentralGoogle Scholar
- Montenegro RD, Oliveira AL, Cabral GG, Katz CR, Rosenblatt A. A comparative study of machine learning techniques for caries prediction. In: 2008 20th IEEE International Conference on tools with artificial intelligence. Piscataway, NJ: IEEE; 2008. p. 477–81. ChapterGoogle Scholar
- Patil S, Habib Awan K, Arakeri G, Jayampath Seneviratne C, Muddur N, Malik S, et al. Machine learning and its potential applications to the genomic study of head and neck cancer—a systematic review. J Oral Pathol Med. 2019;48(9):773–9. ArticlePubMedGoogle Scholar
- Kebschull M, Papapanou PN. Exploring genome-wide expression profiles using machine learning techniques. Methods Oral Biol. 2017;1537:347–64. Springer ArticleGoogle Scholar
- Ritchie MD, Holzinger ER, Li R, Pendergrass SA, Kim D. Methods of integrating data to uncover genotype–phenotype interactions. Nat Rev Genet. 2015;16(2):85–97. ArticlePubMedGoogle Scholar
- Misra BB, Langefeld C, Olivier M, Cox LA. Integrated omics: tools, advances and future approaches. J Mol Endocrinol. 2019;62(1):R21–45. ArticleGoogle Scholar
- Fröhlich H, Patjoshi S, Yeghiazaryan K, Kehrer C, Kuhn W, Golubnitschaja O. Premenopausal breast cancer: potential clinical utility of a multi-omics based machine learning approach for patient stratification. EPMA J. 2018;9(2):175–86. ArticlePubMedPubMed CentralGoogle Scholar
- Divaris K. Fundamentals of precision medicine. Compend Contin Educ Dent. 2017;38(8 Suppl):30–2. PubMedPubMed CentralGoogle Scholar
- Selwitz RH, Ismail AI, Pitts NB. Dental caries. Lancet. 2007;369(9555):51–9. https://doi.org/10.1016/S0140-6736(07)60031-2. ArticlePubMedGoogle Scholar
- Divaris K. Predicting dental caries outcomes in children: a “risky” concept. J Dent Res. 2016;95(3):248–54. https://doi.org/10.1177/0022034515620779. ArticlePubMedGoogle Scholar
- Burne RA, Zeng L, Ahn SJ, Palmer SR, Liu Y, Lefebure T, et al. Progress dissecting the oral microbiome in caries and health. Adv Dent Res. 2012;24(2):77–80. https://doi.org/10.1177/0022034512449462. ArticlePubMedPubMed CentralGoogle Scholar
- Marsh PD. Microbial ecology of dental plaque and its significance in health and disease. Adv Dent Res. 1994;8(2):263–71. https://doi.org/10.1177/08959374940080022001. ArticlePubMedGoogle Scholar
- Nyvad B, Crielaard W, Mira A, Takahashi N, Beighton D. Dental caries from a molecular microbiological perspective. Caries Res. 2013;47(2):89–102. https://doi.org/10.1159/000345367. ArticlePubMedGoogle Scholar
- Falsetta ML, Klein MI, Colonne PM, Scott-Anne K, Gregoire S, Pai CH, et al. Symbiotic relationship between Streptococcus mutants and Candida albicans synergizes virulence of plaque biofilms in vivo. Infect Immun. 2014;82(5):1968–81. https://doi.org/10.1128/IAI.00087-14. ArticlePubMedPubMed CentralGoogle Scholar
- Delisle AL, Guo M, Chalmers NI, Barcak GJ, Rousseau GM, Moineau S. Biology and genome sequence of Streptococcus mutans phage M102AD. Appl Environ Microbiol. 2012;78(7):2264–71. https://doi.org/10.1128/AEM.07726-11. ArticlePubMedPubMed CentralGoogle Scholar
- Divaris K, Joshi A. The building blocks of precision oral health in early childhood: the ZOE 2.0 study. J Public Health Dent. 2018;80(Suppl 1):S31–6. https://doi.org/10.1111/jphd.12303. ArticlePubMedGoogle Scholar
- Ginnis J, Ferreira Zandona AG, Slade GD, Cantrell J, Antonio ME, Pahel BT, et al. Measurement of early childhood Oral health for research purposes: dental caries experience and developmental defects of the enamel in the primary dentition. Methods Mol Biol. 1922;2019:511–23. https://doi.org/10.1007/978-1-4939-9012-2_39. ArticleGoogle Scholar
- Divaris K, Shungin D, Rodriguez-Cortes A, Basta PV, Roach J, Cho H, et al. The Supragingival biofilm in early childhood caries: clinical and laboratory protocols and bioinformatics pipelines supporting metagenomics, Metatranscriptomics, and metabolomics studies of the Oral microbiome. Methods Mol Biol. 1922;2019:525–48. https://doi.org/10.1007/978-1-4939-9012-2_40. ArticleGoogle Scholar
- Haworth S, Esberg A, Lif Holgerson P, Kuja-Halkola R, Timpson NJ, Magnusson PKE, et al. Heritability of caries scores, trajectories, and disease subtypes. J Dent Res. 2020;99(3):264–70. https://doi.org/10.1177/0022034519897910. ArticlePubMedGoogle Scholar
- Shaffer JR, Feingold E, Wang X, Tcuenco KT, Weeks DE, DeSensi RS, et al. Heritable patterns of tooth decay in the permanent dentition: principal components and factor analyses. BMC Oral Health. 2012;12:7. https://doi.org/10.1186/1472-6831-12-7. ArticlePubMedPubMed CentralGoogle Scholar
- GlobalSurg C. Writing g, patient r, statistical a, protocol d, project s, et al. global variation in anastomosis and end colostomy formation following left-sided colorectal resection. BJS Open. 2019;3(3):403–14. https://doi.org/10.1002/bjs5.50138. ArticleGoogle Scholar
- Divaris K. Searching deep and wide: advances in the molecular understanding of dental caries and periodontal disease. Adv Dent Res. 2019;30(2):40–4. https://doi.org/10.1177/0022034519877387. ArticlePubMedPubMed CentralGoogle Scholar
- Wood DE, Salzberg SL. Kraken: ultrafast metagenomic sequence classification using exact alignments. Genome Biol. 2014;15(3):R46. https://doi.org/10.1186/gb-2014-15-3-r46. ArticlePubMedPubMed CentralGoogle Scholar
- Huson DH, Auch AF, Qi J, Schuster SC. MEGAN analysis of metagenomic data. Genome Res. 2007;17(3):377–86. https://doi.org/10.1101/gr.5969107. ArticlePubMedPubMed CentralGoogle Scholar
- Brady A, Salzberg S. PhymmBL expanded: confidence scores, custom databases, parallelization and more. Nat Methods. 2011;8(5):367. https://doi.org/10.1038/nmeth0511-367. ArticlePubMedPubMed CentralGoogle Scholar
- Craig J. Complex diseases: research and applications. Nature Education. 2008;1(1):184. Google Scholar
- The Human Genome Project. https://www.genome.gov/human-genome-project. 2018; Accessed 2020.
- The International HapMap Consortium. The international HapMap project. Nature. 2003;426(6968):789–96. ArticleGoogle Scholar
- The International HapMap Consortium. A haplotype map of the human genome. Nature. 2005;437:1299–320. ArticlePubMed CentralGoogle Scholar
- The International HapMap Consortium. A second generation human haplotype map of over 3.1 million SNPs. Nature. 2007;449:851–61. ArticlePubMed CentralGoogle Scholar
- The International HapMap Consortium. Integrating common and rare genetic variation in diverse human populations. Nature. 2010;467(7311):52–8. https://doi.org/10.1038/nature09298. ArticleGoogle Scholar
- The 1000 Genomes Project Consortium. A map of human genome variation from population-scale sequencing. Nature. 2010;467(7319):1061–73. http://www.nature.com/nature/journal/v467/n7319/abs/nature09534.html#supplementary-informationArticlePubMed CentralGoogle Scholar
- Abecasis GR, Auton A, Brooks LD, DePristo MA, Durbin RM, Handsaker RE, et al. An integrated map of genetic variation from 1,092 human genomes. Nature. 2012;491(7422):56–65. https://doi.org/10.1038/nature11632. ArticlePubMedGoogle Scholar
- The 1000 Genomes Project Consortium. A global reference for human genetic variation. Nature. 2015;526(7571):68–74. https://doi.org/10.1038/nature15393. ArticleGoogle Scholar
- MacArthur J, Bowler E, Cerezo M, Gil L, Hall P, Hastings E, et al. The new NHGRI-EBI catalog of published genome-wide association studies (GWAS catalog). Nucleic Acids Res. 2017;45(D1):D896–d901. https://doi.org/10.1093/nar/gkw1133. ArticlePubMedGoogle Scholar
- Zhang Y, Liu JS. Bayesian inference of epistatic interactions in case-control studies. Nat Genet. 2007;39(9):1167–73. ArticlePubMedGoogle Scholar
- Han B, Chen X-W, Talebizadeh Z. FEPI-MB: identifying SNPs-disease association using a Markov Blanket-based approach. BMC Bioinform. 2011;12(Suppl 12):S3. ArticleGoogle Scholar
- Uppu S, Krishna A, Gopalan RP. A review on methods for detecting SNP interactions in high-dimensional genomic data. IEEE/ACM Trans Comput Biol Bioinform. 2016;15(2):599–612. ArticlePubMedGoogle Scholar
- Jiang R, Tang W, Wu X, Fu W. A random forest approach to the detection of epistatic interactions in case-control studies. BMC Bioinform. 2009;10(1):S65. ArticleGoogle Scholar
- De Lobel L, Geurts P, Baele G, Castro-Giner F, Kogevinas M, Van Steen K. A screening methodology based on random forests to improve the detection of gene–gene interactions. Eur J Hum Genet. 2010;18(10):1127–32. ArticlePubMedPubMed CentralGoogle Scholar
- Yoshida M, Koike A. SNPInterForest: a new method for detecting epistatic interactions. BMC Bioinform. 2011;12(1):469. ArticleGoogle Scholar
- Schwarz DF, König IR, Ziegler A. On safari to random jungle: a fast implementation of random forests for high-dimensional data. Bioinformatics. 2010;26(14):1752–8. ArticlePubMedPubMed CentralGoogle Scholar
- Wu Q, Ye Y, Liu Y, Ng MK. SNP selection and classification of genome-wide SNP data using stratified sampling random forests. IEEE Trans Nanobioscience. 2012;11(3):216–27. ArticlePubMedGoogle Scholar
- Lin HY, Ann Chen Y, Tsai YY, Qu X, Tseng TS, Park JY. TRM: a powerful two-stage machine learning approach for identifying SNP-SNP interactions. Ann Hum Genet. 2012;76(1):53–62. ArticlePubMedGoogle Scholar
- Pan Q, Hu T, Malley JD, Andrew AS, Karagas MR, Moore JH. Supervising random forest using attribute interaction networks. European conference on evolutionary computation, machine learning and data mining in bioinformatics. Berlin: Springer; 2013. p. 104–16. Google Scholar
- Chen SH, Sun J, Dimitrov L, Turner AR, Adams TS, Meyers DA, et al. A support vector machine approach for detecting gene-gene interaction. Genetic Epidemiology: The Official Publication of the International Genetic Epidemiology Society. 2008;32(2):152–67. ArticleGoogle Scholar
- Özgür A, Vu T, Erkan G, Radev DR. Identifying gene-disease associations using centrality on a literature mined gene-interaction network. Bioinformatics. 2008;24(13):i277–i85. ArticlePubMedPubMed CentralGoogle Scholar
- Shen Y, Liu Z, Ott J. Support vector machines with L 1 penalty for detecting gene-gene interactions. Int J Data Min Bioinform. 2012;6(5):463–70. ArticlePubMedGoogle Scholar
- Fang YH, Chiu YF. SVM-based generalized multifactor dimensionality reduction approaches for detecting gene-gene interactions in family studies. Genet Epidemiol. 2012;36(2):88–98. ArticlePubMedGoogle Scholar
- Marvel S, Motsinger-Reif A. Grammatical evolution support vector machines for predicting human genetic disease association. Proceedings of the 14th annual conference companion on Genetic and evolutionary computation 2012. p. 595–8. Google Scholar
- Zhang H, Wang H, Dai Z, Chen M-S, Yuan Z. Improving accuracy for cancer classification with a new algorithm for genes selection. BMC Bioinform. 2012;13(1):298. ArticleGoogle Scholar
- Lin Y, Jeon Y. Random forests and adaptive nearest neighbors. J Am Stat Assoc. 2006;101(474):578–90. https://doi.org/10.1198/016214505000001230. ArticleGoogle Scholar
- Koo CL, Liew MJ, Mohamad MS, Salleh M, Hakim A. A review for detecting gene-gene interactions using machine learning methods in genetic epidemiology. Biomed Res Int. 2013;2013:432375. ArticlePubMedPubMed CentralGoogle Scholar
- Roller E, Ivakhno S, Lee S, Royce T, Tanner S. Canvas: versatile and scalable detection of copy number variants. Bioinformatics. 2016;32(15):2375–7. ArticlePubMedGoogle Scholar
- Ivakhno S, Roller E, Colombo C, Tedder P, Cox AJ. Canvas SPW: calling de novo copy number variants in pedigrees. Bioinformatics. 2018;34(3):516–8. ArticlePubMedGoogle Scholar
- Wang Z, Hormozdiari F, Yang W-Y, Halperin E, Eskin E. CNVeM: copy number variation detection using uncertainty of read mapping. J Comput Biol. 2013;20(3):224–36. ArticlePubMedPubMed CentralGoogle Scholar
- Nguyen HT, Merriman TR, Black MA. The CNVrd2 package: measurement of copy number at complex loci using high-throughput sequencing data. Front Genet. 2014;5:248. ArticlePubMedPubMed CentralGoogle Scholar
- Miller CA, Hampton O, Coarfa C, Milosavljevic A. ReadDepth: a parallel R package for detecting copy number alterations from short sequencing reads. PLoS One. 2011;6(1):e16327. ArticlePubMedPubMed CentralGoogle Scholar
- Aure MR, Vitelli V, Jernström S, Kumar S, Krohn M, Due EU, et al. Integrative clustering reveals a novel split in the luminal a subtype of breast cancer with impact on outcome. Breast Cancer Res. 2017;19(1):44. https://doi.org/10.1186/s13058-017-0812-y. ArticlePubMedPubMed CentralGoogle Scholar
- Karim MR, Rahman A, Jares JB, Decker S, Beyan O. A snapshot neural ensemble method for cancer-type prediction based on copy number variations. Neural Comput & Applic. 2019:1–19. Google Scholar
- AlShibli A, Mathkour H. A shallow convolutional learning network for classification of cancers based on copy number variations. Sensors. 2019;19(19):4207. ArticlePubMed CentralGoogle Scholar
- Fortin J-P, Triche TJ Jr, Hansen KD. Preprocessing, normalization and integration of the Illumina HumanMethylationEPIC array with minfi. Bioinformatics. 2017;33(4):558–60. ArticlePubMedGoogle Scholar
- Robertson KD. DNA methylation and human disease. Nat Rev Genet. 2005;6(8):597–610. ArticlePubMedGoogle Scholar
- Jiang Y, Oldridge DA, Diskin SJ, Zhang NR. CODEX: a normalization and copy number variation detection method for whole exome sequencing. Nucleic Acids Res. 2015;43(6):e39-e. ArticleGoogle Scholar
- Wang K, Li M, Hadley D, Liu R, Glessner J, Grant SF, et al. PennCNV: an integrated hidden Markov model designed for high-resolution copy number variation detection in whole-genome SNP genotyping data. Genome Res. 2007;17(11):1665–74. ArticlePubMedPubMed CentralGoogle Scholar
- Colella S, Yau C, Taylor JM, Mirza G, Butler H, Clouston P, et al. QuantiSNP: an objective Bayes hidden-Markov model to detect and accurately map copy number variation using SNP genotyping data. Nucleic Acids Res. 2007;35(6):2013–25. ArticlePubMedPubMed CentralGoogle Scholar
- Zhang Z, Cheng H, Hong X, Di Narzo AF, Franzen O, Peng S, et al. EnsembleCNV: an ensemble machine learning algorithm to identify and genotype copy number variation using SNP array data. Nucleic Acids Res. 2019;47(7):e39-e. ArticleGoogle Scholar
- Pounraja VK, Jayakar G, Jensen M, Kelkar N, Girirajan S. A machine-learning approach for accurate detection of copy number variants from exome sequencing. Genome Res. 2019;29(7):1134–43. ArticlePubMedPubMed CentralGoogle Scholar
- Poplin R, Chang P-C, Alexander D, Schwartz S, Colthurst T, Ku A, et al. A universal SNP and small-indel variant caller using deep neural networks. Nat Biotechnol. 2018;36(10):983–7. ArticlePubMedGoogle Scholar
- Hill T, Unckless RL. A deep learning approach for detecting copy number variation in next-generation sequencing data. G3: Genes, Genomes, Genetics. 2019;9(11):3575–82. ArticleGoogle Scholar
- Zhang Y, Jin L, Wang B, Hu D, Wang L, Li P, et al. DL-CNV: a deep learning method for identifying copy number variations based on next generation target sequencing. Math Biosci Eng: MBE. 2019;17(1):202–15. ArticlePubMedGoogle Scholar
- Jiang Y, Qiu Y, Minn AJ, Zhang NR. Assessing intratumor heterogeneity and tracking longitudinal and spatial clonal evolutionary history by next-generation sequencing. Proc Natl Acad Sci. 2016;113(37):E5528–E37. ArticlePubMedPubMed CentralGoogle Scholar
- Liu J, Halloran JT, Bilmes JA, Daza RM, Lee C, Mahen EM, et al. Comprehensive statistical inference of the clonal structure of cancer from multiple biopsies. Sci Rep. 2017;7(1):1–13. Google Scholar
- Holder LB, Haque MM, Skinner MK. Machine learning for epigenetics and future medical applications. Epigenetics. 2017;12(7):505–14. ArticlePubMedPubMed CentralGoogle Scholar
- Ni P, Huang N, Zhang Z, Wang D-P, Liang F, Miao Y, et al. DeepSignal: detecting DNA methylation state from Nanopore sequencing reads using deep-learning. Bioinformatics. 2019;35(22):4586–95. ArticlePubMedGoogle Scholar
- Angermueller C, Lee HJ, Reik W, Stegle O. DeepCpG: accurate prediction of single-cell DNA methylation states using deep learning. Genome Biol. 2017;18(1):67. ArticlePubMedPubMed CentralGoogle Scholar
- Zhang W, Spector TD, Deloukas P, Bell JT, Engelhardt BE. Predicting genome-wide DNA methylation using methylation marks, genomic position, and DNA regulatory elements. Genome Biol. 2015;16(1):14. ArticlePubMedPubMed CentralGoogle Scholar
- Zhang G, Huang KC, Xu Z, Tzeng JY, Conneely KN, Guan W, et al. Across-platform imputation of DNA methylation levels incorporating nonlocal information using penalized functional regression. Genet Epidemiol. 2016;40(4):333–40. https://doi.org/10.1002/gepi.21969. ArticlePubMedPubMed CentralGoogle Scholar
- Zeng H, Gifford DK. Predicting the impact of non-coding variants on DNA methylation. Nucleic Acids Res. 2017;45(11):e99-e. ArticleGoogle Scholar
- Capper D, Jones DT, Sill M, Hovestadt V, Schrimpf D, Sturm D, et al. DNA methylation-based classification of central nervous system tumours. Nature. 2018;555(7697):469–74. ArticlePubMedPubMed CentralGoogle Scholar
- Cai Z, Xu D, Zhang Q, Zhang J, Ngai S-M, Shao J. Classification of lung cancer using ensemble-based feature selection and machine learning methods. Mol BioSyst. 2015;11(3):791–800. ArticlePubMedGoogle Scholar
- Wei SH, Balch C, Paik HH, Kim Y-S, Baldwin RL, Liyanarachchi S, et al. Prognostic DNA methylation biomarkers in ovarian cancer. Clin Cancer Res. 2006;12(9):2788–94. ArticlePubMedGoogle Scholar
- Aran D, Sabato S, Hellman A. DNA methylation of distal regulatory sites characterizes dysregulation of cancer genes. Genome Biol. 2013;14(3):R21. ArticlePubMedPubMed CentralGoogle Scholar
- Forcato M, Nicoletti C, Pal K, Livi CM, Ferrari F, Bicciato S. Comparison of computational methods for Hi-C data analysis. Nat Methods. 2017;14(7):679–85. https://doi.org/10.1038/nmeth.4325. ArticlePubMedPubMed CentralGoogle Scholar
- Rao SS, Huntley MH, Durand NC, Stamenova EK, Bochkov ID, Robinson JT, et al. A 3D map of the human genome at kilobase resolution reveals principles of chromatin looping. Cell. 2014;159(7):1665–80. ArticlePubMedPubMed CentralGoogle Scholar
- Bonev B, Mendelson Cohen N, Szabo Q, Fritsch L, Papadopoulos GL, Lubling Y, et al. Multiscale 3D genome rewiring during mouse neural development. Cell. 2017;171(3):557–72.e24. https://doi.org/10.1016/j.cell.2017.09.043. ArticlePubMedPubMed CentralGoogle Scholar
- Jin F, Li Y, Dixon JR, Selvaraj S, Ye Z, Lee AY, et al. A high-resolution map of the three-dimensional chromatin interactome in human cells. Nature. 2013;503(7475):290–4. https://doi.org/10.1038/nature12644. ArticlePubMedPubMed CentralGoogle Scholar
- Zhang Y, An L, Xu J, Zhang B, Zheng WJ, Hu M, et al. Enhancing Hi-C data resolution with deep convolutional neural network HiCPlus. Nat Commun. 2018;9(1):750. https://doi.org/10.1038/s41467-018-03113-2. ArticlePubMedPubMed CentralGoogle Scholar
- Liu T, Wang Z. HiCNN: a very deep convolutional neural network to better enhance the resolution of Hi-C data. Bioinformatics. 2019;35(21):4222–8. https://doi.org/10.1093/bioinformatics/btz251. ArticlePubMedPubMed CentralGoogle Scholar
- Liu Q, Lv H, Jiang R. hicGAN infers super resolution Hi-C data with generative adversarial networks. Bioinformatics. 2019;35(14):i99–i107. https://doi.org/10.1093/bioinformatics/btz317. ArticlePubMedPubMed CentralGoogle Scholar
- Lajoie BR, Dekker J, Kaplan N. The Hitchhiker’s guide to Hi-C analysis: practical guidelines. Methods. 2015;72:65–75. https://doi.org/10.1016/j.ymeth.2014.10.031. ArticlePubMedGoogle Scholar
- Yaffe E, Tanay A. Probabilistic modeling of Hi-C contact maps eliminates systematic biases to characterize global chromosomal architecture. Nat Genet. 2011;43(11):1059–65. https://doi.org/10.1038/ng.947. ArticlePubMedGoogle Scholar
- Hu M, Deng K, Selvaraj S, Qin Z, Ren B, Liu JS. HiCNorm: removing biases in Hi-C data via Poisson regression. Bioinformatics. 2012;28(23):3131–3. https://doi.org/10.1093/bioinformatics/bts570. ArticlePubMedPubMed CentralGoogle Scholar
- Imakaev M, Fudenberg G, McCord RP, Naumova N, Goloborodko A, Lajoie BR, et al. Iterative correction of Hi-C data reveals hallmarks of chromosome organization. Nat Methods. 2012;9(10):999–1003. https://doi.org/10.1038/nmeth.2148. ArticlePubMedPubMed CentralGoogle Scholar
- Li Y, Hu M, Shen Y. Gene regulation in the 3D genome. Hum Mol Genet. 2018;27(R2):R228–r33. https://doi.org/10.1093/hmg/ddy164. ArticlePubMedPubMed CentralGoogle Scholar
- Yu M, Ren B. The three-dimensional Organization of Mammalian Genomes. Annu Rev Cell Dev Biol. 2017;33:265–89. https://doi.org/10.1146/annurev-cellbio-100616-060531. ArticlePubMedPubMed CentralGoogle Scholar
- Crowley C, Yang Y, Qiu Y, Hu B, Won H, Ren B, et al. FIREcaller: an R package for detecting frequently interacting regions from Hi-C data. bioRxiv. 2019; 619288. https://doi.org/10.1101/619288.
- Schmitt AD, Hu M, Jung I, Xu Z, Qiu Y, Tan CL, et al. A compendium of chromatin contact maps reveals spatially active regions in the human genome. Cell Rep. 2016;17(8):2042–59. https://doi.org/10.1016/j.celrep.2016.10.061. ArticlePubMedPubMed CentralGoogle Scholar
- Rao Suhas SP, Huntley Miriam H, Durand Neva C, Stamenova Elena K, Bochkov Ivan D, Robinson James T, et al. A 3D map of the human genome at kilobase resolution reveals principles of chromatin looping. Cell. 2014;159(7):1665–80. https://doi.org/10.1016/j.cell.2014.11.021. ArticlePubMedPubMed CentralGoogle Scholar
- Kaul A, Bhattacharyya S, Ay F. Identifying statistically significant chromatin contacts from Hi-C data with FitHiC2. Nat Protoc. 2020;15(3):991–1012. https://doi.org/10.1038/s41596-019-0273-0. ArticlePubMedPubMed CentralGoogle Scholar
- Ay F, Bailey TL, Noble WS. Statistical confidence estimation for Hi-C data reveals regulatory chromatin contacts. Genome Res. 2014; https://doi.org/10.1101/gr.160374.113.
- Juric I, Yu M, Abnousi A, Raviram R, Fang R, Zhao Y, et al. MAPS: model-based analysis of long-range chromatin interactions from PLAC-seq and HiChIP experiments. PLoS Comput Biol. 2019;15(4):e1006982. https://doi.org/10.1371/journal.pcbi.1006982. ArticlePubMedPubMed CentralGoogle Scholar
- Xu Z, Zhang G, Jin F, Chen M, Furey TS, Sullivan PF, et al. A hidden Markov random field-based Bayesian method for the detection of long-range chromosomal interactions in Hi-C data. Bioinformatics. 2016;32(5):650–6. https://doi.org/10.1093/bioinformatics/btv650. ArticlePubMedGoogle Scholar
- Xu Z, Zhang G, Wu C, Li Y, Hu M. FastHiC: a fast and accurate algorithm to detect long-range chromosomal interactions from Hi-C data. Bioinformatics. 2016;32(17):2692–5. https://doi.org/10.1093/bioinformatics/btw240. ArticlePubMedPubMed CentralGoogle Scholar
- Ay F, Bailey TL, Noble WS. Statistical confidence estimation for Hi-C data reveals regulatory chromatin contacts. Genome Res. 2014;24(6):999–1011. https://doi.org/10.1101/gr.160374.113. ArticlePubMedPubMed CentralGoogle Scholar
- Lawrence CE, Reilly AA. An expectation maximization (EM) algorithm for the identification and characterization of common sites in unaligned biopolymer sequences. Proteins. 1990;7(1):41–51. https://doi.org/10.1002/prot.340070105. ArticlePubMedGoogle Scholar
- Bailey TL, Williams N, Misleh C, Li WW. MEME: discovering and analyzing DNA and protein sequence motifs. Nucleic Acids Res. 2006;34(Web Server issue):W369–73. https://doi.org/10.1093/nar/gkl198. ArticlePubMedPubMed CentralGoogle Scholar
- Moses AM, Chiang DY, Eisen MB. Phylogenetic motif detection by expectation-maximization on evolutionary mixtures. Pac Symp Biocomput. 2004:324–35. https://doi.org/10.1142/9789812704856_0031.
- Prakash A, Blanchette M, Sinha S, Tompa M. Motif discovery in heterogeneous sequence data. Pac Symp Biocomput. 2004:348–59. https://doi.org/10.1142/9789812704856_0033.
- Sinha S, Blanchette M, Tompa M. PhyME: a probabilistic algorithm for finding motifs in sets of orthologous sequences. BMC Bioinform. 2004;5:170. https://doi.org/10.1186/1471-2105-5-170. ArticleGoogle Scholar
- Alipanahi B, Delong A, Weirauch MT, Frey BJ. Predicting the sequence specificities of DNA- and RNA-binding proteins by deep learning. Nat Biotechnol. 2015;33(8):831–8. https://doi.org/10.1038/nbt.3300. ArticlePubMedGoogle Scholar
- Machanick P, Bailey TL. MEME-ChIP: motif analysis of large DNA datasets. Bioinformatics. 2011;27(12):1696–7. ArticlePubMedPubMed CentralGoogle Scholar
- Foat BC, Morozov AV, Bussemaker HJ. Statistical mechanical modeling of genome-wide transcription factor occupancy data by MatrixREDUCE. Bioinformatics. 2006;22(14):e141–e9. ArticlePubMedGoogle Scholar
- Quang D, Xie X. FactorNet: a deep learning framework for predicting cell type specific transcription factor binding from nucleotide-resolution sequential data. Methods. 2019;166:40–7. https://doi.org/10.1016/j.ymeth.2019.03.020. ArticlePubMedPubMed CentralGoogle Scholar
- Zhou J, Troyanskaya OG. Predicting effects of noncoding variants with deep learning-based sequence model. Nat Methods. 2015;12(10):931–4. https://doi.org/10.1038/nmeth.3547. ArticlePubMedPubMed CentralGoogle Scholar
- Ritchie GR, Dunham I, Zeggini E, Flicek P. Functional annotation of noncoding sequence variants. Nat Methods. 2014;11(3):294–6. https://doi.org/10.1038/nmeth.2832. ArticlePubMedPubMed CentralGoogle Scholar
- Wang M, Tai C, Weinan E, Wei L. DeFine: deep convolutional neural networks accurately quantify intensities of transcription factor-DNA binding and facilitate evaluation of functional non-coding variants. Nucleic Acids Res. 2018;46(11):e69. https://doi.org/10.1093/nar/gky215. ArticlePubMedPubMed CentralGoogle Scholar
- Stuart T, Butler A, Hoffman P, Hafemeister C, Papalexi E, Mauck WM III, et al. Comprehensive integration of single-cell data. Cell. 2019;177(7):1888–1902.e21. ArticlePubMedPubMed CentralGoogle Scholar
- Adey AC. Integration of single-cell genomics datasets. Cell. 2019;177(7):1677–9. ArticlePubMedGoogle Scholar
- Welch JD, Kozareva V, Ferreira A, Vanderburg C, Martin C, Macosko EZ. Single-cell multi-omic integration compares and contrasts features of brain cell identity. Cell. 2019;177:1873–1887.e17. ArticlePubMedPubMed CentralGoogle Scholar
- Li G, Yang Y, Van Buren E, Li Y. Dropout imputation and batch effect correction for single-cell RNA sequencing data. J Bio-X Res. 2019;2(4):169–77. Google Scholar
- Bengio Y. Learning deep architectures for AI. Foundations and trends® in. Mach Learn. 2009;2(1):1–127. Google Scholar
- Zhang X, Zhao J, LeCun Y. Character-level convolutional networks for text classification. Adv Neural Inform Proc Syst. 2015:649–57. Google Scholar
- Deng Y, Bao F, Dai Q, Wu LF, Altschuler SJ. Scalable analysis of cell-type composition from single-cell transcriptomics using deep recurrent learning. Nat Methods. 2019;16(4):311–4. ArticlePubMedPubMed CentralGoogle Scholar
- Lopez R, Regier J, Cole MB, Jordan MI, Yosef N. Deep generative modeling for single-cell transcriptomics. Nat Methods. 2018;15(12):1053–8. ArticlePubMedPubMed CentralGoogle Scholar
- Van Dijk D, Sharma R, Nainys J, Yim K, Kathail P, Carr AJ, et al. Recovering gene interactions from single-cell data using data diffusion. Cell. 2018;174(3):716–729.e27. ArticlePubMedPubMed CentralGoogle Scholar
- Eraslan G, Simon LM, Mircea M, Mueller NS, Theis FJ. Single-cell RNA-seq denoising using a deep count autoencoder. Nat Commun. 2019;10(1):1–14. ArticleGoogle Scholar
- Way GP, Greene CS. Bayesian deep learning for single-cell analysis. Nat Methods. 2018;15(12):1009–10. ArticlePubMedGoogle Scholar
- Goodfellow I, Pouget-Abadie J, Mirza M, Xu B, Warde-Farley D, Ozair S, et al. Generative adversarial nets. Adv Neural Inform Process Syst. 2014;3:2672–80. Google Scholar
- Wolf FA, Angerer P, Theis FJ. SCANPY: large-scale single-cell gene expression data analysis. Genome Biol. 2018;19(1):15. ArticlePubMedPubMed CentralGoogle Scholar
- Satija R, Farrell JA, Gennert D, Schier AF, Regev A. Spatial reconstruction of single-cell gene expression data. Nat Biotechnol. 2015;33(5):495–502. ArticlePubMedPubMed CentralGoogle Scholar
- Trapnell C, Cacchiarelli D, Grimsby J, Pokharel P, Li S, Morse M, et al. The dynamics and regulators of cell fate decisions are revealed by pseudotemporal ordering of single cells. Nat Biotechnol. 2014;32(4):381. ArticlePubMedPubMed CentralGoogle Scholar
- Kharchenko PV, Silberstein L, Scadden DT. Bayesian approach to single-cell differential expression analysis. Nat Methods. 2014;11(7):740. ArticlePubMedPubMed CentralGoogle Scholar
- Finak G, McDavid A, Yajima M, Deng J, Gersuk V, Shalek AK, et al. MAST: a flexible statistical framework for assessing transcriptional changes and characterizing heterogeneity in single-cell RNA sequencing data. Genome Biol. 2015;16(1):278. ArticlePubMedPubMed CentralGoogle Scholar
- Zheng GX, Terry JM, Belgrader P, Ryvkin P, Bent ZW, Wilson R, et al. Massively parallel digital transcriptional profiling of single cells. Nat Commun. 2017;8(1):1–12. ArticleGoogle Scholar
- Lun AT, McCarthy DJ, Marioni JC. A step-by-step workflow for low-level analysis of single-cell RNA-seq data with Bioconductor. F1000Research. 2016;5:2122. PubMedPubMed CentralGoogle Scholar
- Chen W-P, Chang S-H, Tang C-Y, Liou M-L, Tsai S-JJ, Lin Y-L. Composition analysis and feature selection of the oral microbiota associated with periodontal disease. Biomed Res Int. 2018 Google Scholar
- Nakano Y, Suzuki N, Kuwata F. Predicting oral malodour based on the microbiota in saliva samples using a deep learning approach. BMC Oral Health. 2018;18(1):128. ArticlePubMedPubMed CentralGoogle Scholar
- Hsieh C-H, Chen W-M, Hsieh Y-S, Fan Y-C, Yang PE, Kang S-T, et al. A novel multi-gene detection platform for the analysis of miRNA expression. Sci Rep. 2018;8(1):1–9. ArticleGoogle Scholar
- Saxena D, Caufield PW, Li Y, Brown S, Song J, Norman R. Genetic classification of severe early childhood caries by use of subtracted DNA fragments from Streptococcus mutans. J Clin Microbiol. 2008;46(9):2868–73. ArticlePubMedPubMed CentralGoogle Scholar
- Carnielli CM, Macedo CCS, De Rossi T, Granato DC, Rivera C, Domingues RR, et al. Combining discovery and targeted proteomics reveals a prognostic signature in oral cancer. Nat Commun. 2018;9(1):1–17. ArticleGoogle Scholar
- Torres PJ, Thompson J, McLean JS, Kelley ST, Edlund A. Discovery of a novel periodontal disease-associated bacterium. Microb Ecol. 2019;77(1):267–76. ArticlePubMedGoogle Scholar
- Vapnik V. The nature of statistical learning theory. Berlin: Springer Science & Business Media; 2000. BookGoogle Scholar
- Kramer MA. Nonlinear principal component analysis using autoassociative neural networks. AICHE J. 1991;37(2):233–43. ArticleGoogle Scholar
- Oh M, Zhang L. DeepMicro: deep representation learning for disease prediction based on microbiome data. Sci Rep. 2020;10(1):1–9. Google Scholar
- Reiman D, Metwally A, Dai Y, Sun J. PopPhy-CNN: a phylogenetic tree embedded architecture for convolutional neural networks to predict host phenotype from metagenomic data. IEEE J Biomed Health Inform. 2020;24(10):2993–3001. ArticlePubMedGoogle Scholar
Acknowledgments
This work was supported by grants from the National Institutes of Health (NIH), National Institute of Dental and Craniofacial Research, R03-DE028983 to DW and HC, U01-DE025046 to KD and HC, NIH R01 GM105785, R01 HL129132, and R01 HL146500 to YL, and NLM T15-LM012500 to MP.
Author information
Authors and Affiliations
- Division of Oral and Craniofacial Health Science, Adam School of Dentistry, University of North Carolina at Chapel Hill, Chapel Hill, NC, USA Di Wu
- Department of Biostatistics, Gillings School of Global Public Health, University of North Carolina at Chapel Hill, Chapel Hill, NC, USA Di Wu & Hunyong Cho
- Division of Pediatric and Public Health, Adam School of Dentistry, University of North Carolina at Chapel Hill, Chapel Hill, NC, USA Deepti S. Karhade & Kimon Divaris
- Carolina Health Informatics Program, University of North Carolina at Chapel Hill, Chapel Hill, NC, USA Malvika Pillai
- Department of Applied Physical Sciences, University of North Carolina at Chapel Hill, Chapel Hill, NC, USA Min-Zhi Jiang
- Department of Genetics, University of North Carolina at Chapel Hill, Chapel Hill, NC, USA Le Huang & Yun Li
- Department of Statistics and Operations Research, University of North Carolina-Chapel Hill, Chapel Hill, USA Gang Li
- Research Computing, University of North Carolina-Chapel Hill, Chapel Hill, USA Jeff Roach
- Department of Epidemiology, Gillings School of Global Public Health, University of North Carolina-Chapel Hill, Chapel Hill, USA Kimon Divaris